Abstract: This paper co
nsiders a three-dimensio
nal latent factor model in the presence of one set of global factors and two sets of local factors. We allow the numbers of local factors to vary across individuals and show that the numbers of global and local factors can be estimated uniformly consistently. Given the number of global and local factors, we propose a two-step estimation procedure ba
sed on principal compo
nent analysis (PCA). Our first step estimates the global factors and their factor loadings, after which we estimate the two sets of local factors and factor loadings sequentially. Our second step improves the estimation efficiency. The asymptotic theories for our estimators are established. Mo
nte Carlo simulations demo
nstrate that they perform well in finite samples. Applications to two datasets in internatio
nal trade and eco
nomic growth reveal the relative im
portance of different types of factors. In the internatio
nal trade application, we find that the global factors, source country factors, and destination country factors are all im
portant. In the industrial growth application, there is no global factor and the country factors are far more im
portant than the industry factors. The extension to the 3D factor model with covariates is also studied.
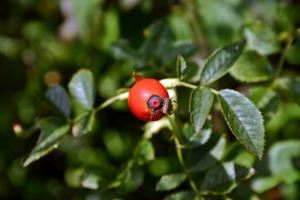